In January 2016, I joined Google X, Alphabet’s secretive innovation lab. My role was to help manage the people and technology from the nine robotics companies that Google had acquired. There was uncertainty among the team, as Andy Rubin, known as the “father of Android,” had abruptly left without a clear explanation. Larry Page and Sergey Brin occasionally provided some guidance, though it was sporadic due to their busy schedules. Astro Teller, the head of Google X, had agreed a few months earlier to bring all the robotics teams under one roof, affectionately called the “moonshot factory.”
I was drawn to Google X because Astro convinced me it was different from other corporate innovation labs. With a focus on thinking big and backed by patient capital, it seemed like the perfect environment for bold ideas. After years of launching and selling tech startups, this felt like a place where I could work on projects with the potential to change the world. One of those audacious goals was developing AI-powered robots to live and work alongside humans.
Eight and a half years later, and 18 months after Google decided to stop its biggest bet on robotics and AI, a new robotics startup seems to emerge weekly. Despite the market’s enthusiasm, I worry that Silicon Valley, with its focus on quick solutions and venture capitalists’ reluctance to invest in hardware, may lack the patience needed to win the global race to integrate AI with robotics. Much of the investment is focused on the wrong areas, and here’s why.
The Concept of a “Moonshot”
Google X, later home to the project known as Everyday Robots, was established in 2010 with the ambitious goal of addressing the world’s toughest problems. The lab was located away from Google’s main campus, allowing it to cultivate a culture of risk-taking and innovation. The ethos at X was about taking big risks, experimenting quickly, and even celebrating failure, which was seen as a sign of aiming high. When I joined, the lab had already birthed projects like Waymo, Google Glass, and other futuristic endeavors such as flying wind turbines and stratospheric balloons for providing internet access.
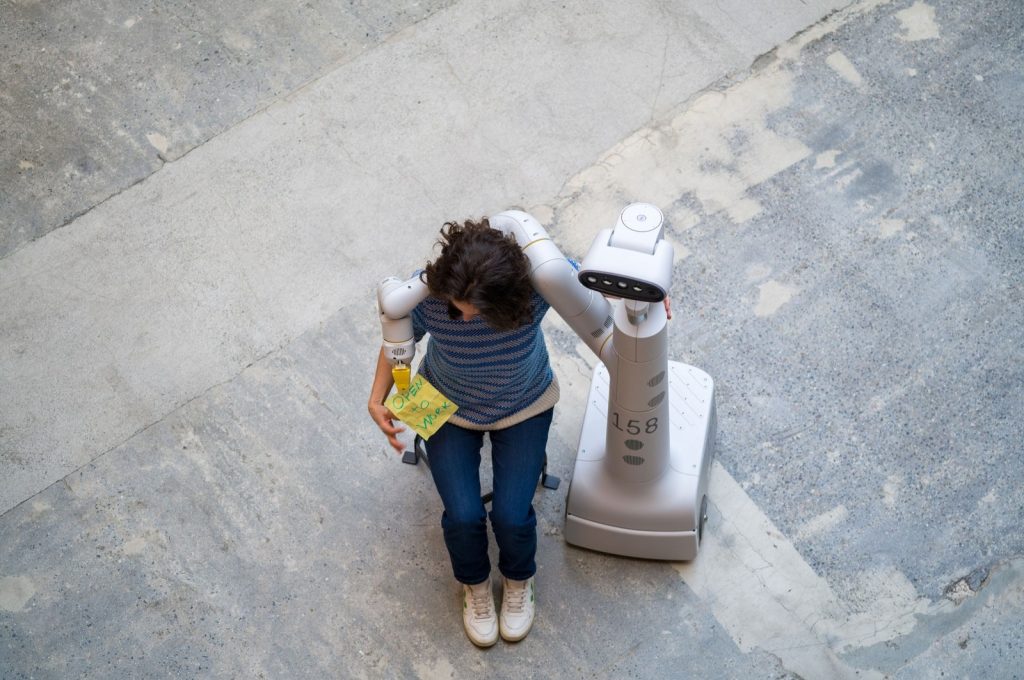
What set X apart from Silicon Valley startups was the scale and long-term thinking encouraged by the leadership. For a project to qualify as a “moonshot,” it had to meet three criteria. First, it needed to address a problem that impacted hundreds of millions or even billions of people. Second, there had to be a breakthrough technology offering a new way to solve the problem. Finally, the solution had to be radical—so bold that it might initially sound outlandish.
The AI and Robotics Challenge
Astro Teller, X’s “Captain of Moonshots,” was uniquely suited to lead this endeavor. Always seen wearing rollerblades and a friendly smile, Astro embodied the spirit of big thinking and bold ideas. When we first sat down to discuss what to do with Google’s acquired robot companies, we knew we had to find a new approach. Up to that point, most robots were large, unintelligent, and dangerous, primarily confined to factories where they required heavy supervision or isolation from humans. Our challenge was to create robots that could safely and effectively function in everyday environments.
We were tackling a global issue—the aging population, shrinking workforces, and labor shortages. Our breakthrough technology was artificial intelligence, which we already believed in 2016 would be crucial to building fully autonomous robots that could assist with a growing list of tasks in daily life.
In essence, we were trying to give AI a body in the physical world. I believed that if any place could accomplish this, it was X. However, we knew it would take time, patience, and a willingness to embrace failure. The technical breakthroughs required for AI and robot technology would likely cost billions of dollars. But the team had a deep conviction that the convergence of AI and robotics was inevitable. What had long been confined to science fiction was about to become reality.
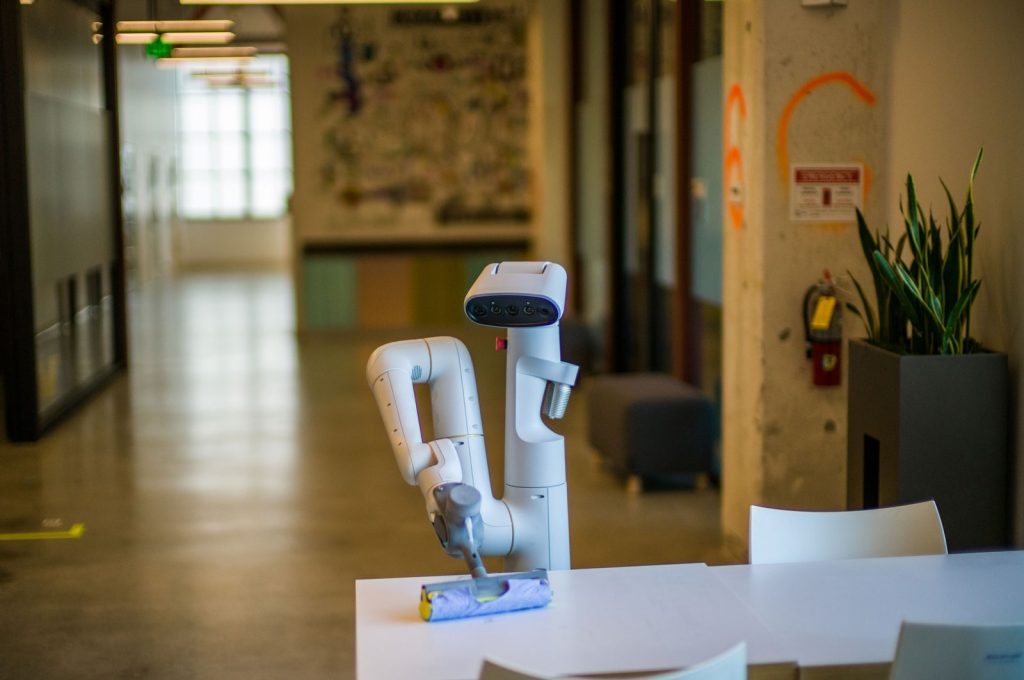
Challenges in Robotics
One of my weekly conversations with my mother always started with the same question: “When are the robots coming?” Living in Oslo, she relied on public healthcare workers to assist with tasks related to her Parkinson’s disease. While grateful for the help, she dreamed of robots that could provide the extra support she needed. I would tell her, “It’ll be a while, Mom,” but she was always eager for progress.
The complexity of robotics, as explained by my colleague Jeff Bingham, lies in its system-wide challenges. Robots are only as effective as their weakest components. For instance, if a robot’s vision system struggles in direct sunlight, it might become “blind” when exposed to it. If it can’t navigate stairs, it could fall and cause harm. Building a robot that can function in the unpredictable real world is incredibly difficult.
Decades of attempts to program robots to perform basic tasks, like picking up a cup, have consistently failed due to the unpredictability of real-world conditions. This is why factory robots work in controlled environments, where lighting and object placement are predictable, and they don’t have to worry about human interference.
End-to-End Learning
Larry Page once told me that all we needed were 17 machine-learning experts to succeed in robotics. While I initially thought the number was arbitrary, I later realized his point: real breakthroughs come from small, focused teams, not armies of engineers. The key was in using end-to-end learning (e2e), where AI learns entire tasks, like picking up objects or tidying a room, through exposure to large amounts of data. This approach mimics how humans learn physical tasks.
In the lab, we had what we called the “arm-farm,” where robotic arms repeatedly tried to pick up various objects. Initially, they had a low success rate, but through reinforcement learning, they improved. Watching a robot arm nudge objects out of the way to pick up a specific item marked a significant turning point. The robot wasn’t following pre-programmed instructions—it had learned how to achieve the task.
This progress demonstrated that AI-powered robots could learn to perform tasks in messy, unpredictable environments, moving us closer to the goal of creating fully autonomous robots that could assist us in everyday life.